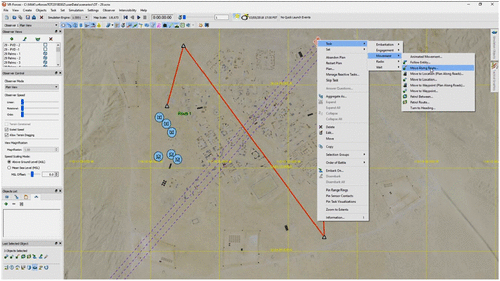
Estimating the tactical impact of robot swarms using VR-Forces
This article was written in collaboration with Dr. Kevin Foster at the University of Alabama in Huntsville.
Militaries are developing swarms of autonomous robotic Unmanned Aircraft Systems (UAS) to conduct missions that are too hazardous for human crews or expensive robotic systems. Using MAK's VR-Forces CGF, researchers at the University of Alabama in Huntsville (UAH) are conducting experiments to determine if a simulated model of a robot swarm can improve military swarm tactics.
Robot swarms (groups of robots acting autonomously to achieve a common goal) conduct complex missions using many simple, inexpensive robots. Defined by basic rules that govern interactions between the robots in the swarm and environmental influences, the swarm uses tactics and control schemes to generate coordination and emergent behavior through swarm intelligence.
Because operational robot swarms are not yet available in the real world, doctrine developers use constructive entity-level combat models, like MAK's VR-Forces, to devise employment tactics for robot swarms. Here's how UAH is doing it.
UAH's Robot Swarm Experiments Using VR-Forces
Over the last two years, UAH researchers Dr. Mikel Petty and Dr. Kevin Foster researched models of swarm robots as a part of Dr. Foster's dissertation work. The research included three integrated efforts:
- Use Design of Experiments (DoE) methods and retrodiction of a historical battle to calibrate model parameter settings and estimate the tactical impact of a simple robot swarm in VR-Forces – we'll discuss this effort below.
- Develop an autonomous robot swarm model in VR-Forces
- Improve the tactical performance of the swarm using a genetic algorithm implemented with VR-Forces Lua scripts
The rest of this blog post covers the first effort listed above. The results were published in the July 2021 issue of the Journal of Defense Modeling and Simulation, titled "Estimating the tactical impact of robot swarms using a semi-automated forces system and design of experiments methods." The combat model was calibrated using retroactive prediction, or ''retrodiction,'' a method that involves simulating a historical battle and comparing the simulation results to the battle's historical outcome. For this experiment, the researchers used the Battle of 73 Easting as the historical battle to simulate in VR-Forces. The calibrated combat model was then used to estimate the tactical impact of a notional Iraqi robot swarm conducting reconnaissance and surveillance in that battle.
The Battle of 73 Easting, which was influential in the overall outcome of the 1991 Gulf War, was fought on 26 February 1991 in featureless desert terrain near the Iraq–Kuwait border. It was fought between the 2nd Armored Cavalry Regiment (2ACR) of the United States Army and two brigades of the Tawakalna Division of the Iraqi Republican Guard. An extensive analysis of the battlefield and interviews with participants in the weeks after the Battle of 73 Easting provided the information needed to create and validate a VR-Forces model of the battle.
The Design of Experiments methods produced estimates of the interaction of the robot swarm's effect with the technologies of the combatants' weapon systems. Simulation trials and statistical analysis showed that the tactical benefits of an Iraqi robot swarm were overshadowed by the advantage provided by the US forces' thermal sights. However, additional trials indicated that if both sides had been equipped with optical sights only, the early warning provided to the Iraqi forces by a robot swarm could have had a significant effect on the battle's outcome.
"Using VR-Forces was an integral piece of our experiments to understand how to model robot swarms using a CGF system and how that model could be used to improve swarm tactics, " said Dr. Kevin Foster. "VR-Forces provides an extensive set of advanced entity models and high-resolution terrain databases that facilitate the development of robot swarm models that operate in complex urban terrain. The VR-Forces Lua scripting language interface provides an efficient method to modify existing entity models to exhibit autonomous behavior and develop a flexible genetic algorithm to improve swarm tactics. With VR-Forces' capability for batch execution, the machine learning model we developed enhanced swarm tactics over hundreds of unattended simulation scenarios."
Additional research papers documenting the autonomous swarm model and the genetic algorithm to improve swarm tactical performance are under development and will be available in the coming months – stay tuned!